Databricks is arguably an ideal candidate governing data, developing machine learning models and performing experiments. The interactive and collaborative nature of the platform makes it easy for engineers and scientists to work together, deliver solutions and gain insights of complex data collections. Machine learning models need to be constantly updated and adapt to new data. Also, it is important to keep track of historical performance of such models and even roll back to previous versions. In this article we will explore how MLflow can help us streamline and automate some common tasks that data practitioners face on a daily basis, and why Databricks paired with MLflow is a great combination for tackling problems in the field of MLOps.
What is MLflow
MLflow is an open-source platform that helps streamline and manage the end-to-end machine learning lifecycle, providing tools for experimentation, reproducibility, and deployment. Some of the key features include
- Experiment Tracking where parameters, metrics, and artifacts from experiments are logged, making it easy to compare results across models and experiments.
- Model Management where registration, versioning, and managing the machine learning models life cycle is supported from development to production.
- Models can be deployed in various environments such as cloud, local, or Docker, with support for multiple frameworks.
- Seamless integration with popular ML libraries like TensorFlow, PyTorch, and scikit-learn, allowing flexible workflow integration.
Databricks & MLflow end-to-end example
We will develop a Python notebook and present a typical life cycle of a machine learning project with the help of Databricks and MLflow. The end goal is to build a model that predicts the quality of wine based on several properties like acidity, pH, alcohol, etc.
First of, we load the data from Unity catalog as pandas Dataframes
import pandas as pd
CATALOG_NAME = "nextlytics"
SCHEMA_NAME = "nextlytics-demo"
white_wine = spark.table(f"{CATALOG_NAME}.`{SCHEMA_NAME}`.winequality_red").toPandas()
red_wine = spark.table(f"{CATALOG_NAME}.`{SCHEMA_NAME}`.winequality_white").toPandas()
red_wine['is_red'] = 1
white_wine['is_red'] = 0
data = pd.concat([red_wine, white_wine], axis=0)
# Remove spaces from column names
data.rename(columns=lambda x: x.replace(' ', '_'), inplace=True)
# Consider a wine’s quality as high, if it’s 7 or above
high_quality = (data.quality >= 7).astype(int)
data.quality = high_quality
The final dataset is the following.
We can now split the dataset into training, validation and testing subsets. 60% of the total data will be used for training, 20% for validation and 20% for testing.
from sklearn.model_selection import train_test_split
X = data.drop(["quality"], axis=1)
y = data.quality
# Split out the training data
X_train, X_rem, y_train, y_rem = train_test_split(
X, y, train_size=0.6, random_state=123
)
# Split the remaining data equally into validation and test
X_val, X_test, y_val, y_test = train_test_split(
X_rem, y_rem, test_size=0.5, random_state=123
)
This task seems well suited to a random forest classifier, since the output is binary and there may be interactions between multiple variables. We build the model, and track its performance using the accuracy score.
import mlflow.pyfunc
import mlflow.sklearn
import numpy as np
import sklearn
from sklearn.ensemble import RandomForestClassifier
from sklearn.metrics import roc_auc_score, accuracy_score
from mlflow.models.signature import infer_signature
from mlflow.utils.environment import _mlflow_conda_env
import cloudpickle
import time
# The predict method of sklearn's RandomForestClassifier returns a binary classification (0 or 1).
# The following code creates a wrapper function, SklearnModelWrapper, that uses
# the predict_proba method to return the probability that the observation belongs to each class.
class SklearnModelWrapper(mlflow.pyfunc.PythonModel):
def __init__(self, model):
self.model = model
def predict(self, context, model_input):
return self.model.predict_proba(model_input)[:, 1]
# mlflow.start_run creates a new MLflow run to track the performance of this model.
# Within the context, you call mlflow.log_param to keep track of the parameters used, and
# mlflow.log_metric to record metrics like accuracy.
with mlflow.start_run(run_name="untuned_random_forest"):
n_estimators = 10
model = RandomForestClassifier(
n_estimators=n_estimators, random_state=np.random.RandomState(123)
)
model.fit(X_train, y_train)
# predict_proba returns [prob_negative, prob_positive], so slice the output with [:, 1]
predictions_test = model.predict_proba(X_test)[:, 1]
# auc_score = roc_auc_score(y_test, predictions_test)
accuracy = accuracy_score(y_test, predictions_test > 0.5)
mlflow.log_param("n_estimators", n_estimators)
# Use accuracy as a metric.
mlflow.log_metric("accuracy", accuracy)
wrappedModel = SklearnModelWrapper(model)
# Log the model with a signature that defines the schema of the model's inputs and outputs.
# When the model is deployed, this signature will be used to validate inputs.
signature = infer_signature(X_train, wrappedModel.predict(None, X_train))
# MLflow contains utilities to create a conda environment used to serve models.
# The necessary dependencies are added to a conda.yaml file which is logged along with the model.
conda_env = _mlflow_conda_env(
additional_conda_deps=None,
additional_pip_deps=[
"cloudpickle=={}".format(cloudpickle.__version__),
"scikit-learn=={}".format(sklearn.__version__),
],
additional_conda_channels=None,
)
mlflow.pyfunc.log_model(
"random_forest_model",
python_model=wrappedModel,
conda_env=conda_env,
signature=signature,
)
We can now register the model to Unity catalog and reference it anywhere in Databricks.
run_id = (
mlflow.search_runs(filter_string='tags.mlflow.runName = "untuned_random_forest"')
.iloc[0]
.run_id
)
# Register the model to Unity Catalog.
model_name = f"{CATALOG_NAME}.{SCHEMA_NAME}.wine_quality"
model_version = mlflow.register_model(f"runs:/{run_id}/random_forest_model", model_name)
The model will appear in Unity catalog, under the “Models” section.
From there, we can see some details about the current version of the model.
How your business benefits from
Artificial Intelligence and Machine Learning -
Download the Whitepaper here!
We can now retrieve the model by specifying the version and use it to generate predictions again.
version = 1
model = mlflow.pyfunc.load_model(f"models:/{model_name}/{version}")
# Sanity-check: This should match the accuracy logged by MLflow
print(f'Accuracy: {accuracy_score(y_test, model.predict(X_test) > 0.5)}')
The random forest model performed well even without hyperparameter tuning. The accuracy is over 86% but there’s always room for improvement. We can now use the powerful XGBoost library in order to run a detailed hyperparameter optimization for the model. In this experiment, we try to maximize the area under the curve score (AUC).
from hyperopt import fmin, tpe, hp, SparkTrials, Trials, STATUS_OK
from hyperopt.pyll import scope
from math import exp
import mlflow.xgboost
import numpy as np
import xgboost as xgb
search_space = {
'max_depth': scope.int(hp.quniform('max_depth', 4, 100, 1)),
'learning_rate': hp.loguniform('learning_rate', -3, 0),
'reg_alpha': hp.loguniform('reg_alpha', -5, -1),
'reg_lambda': hp.loguniform('reg_lambda', -6, -1),
'min_child_weight': hp.loguniform('min_child_weight', -1, 3),
'objective': 'binary:logistic',
'seed': 123, # Set a seed for deterministic training
}
def train_model(params):
# With MLflow autologging, hyperparameters and the trained model are automatically logged to MLflow.
mlflow.xgboost.autolog()
with mlflow.start_run(nested=True):
train = xgb.DMatrix(data=X_train, label=y_train)
validation = xgb.DMatrix(data=X_val, label=y_val)
# Pass in the validation set so xgb can track an evaluation metric. XGBoost terminates training when the evaluation metric
# is no longer improving.
booster = xgb.train(params=params, dtrain=train, num_boost_round=1000,\
evals=[(validation, "validation")], early_stopping_rounds=50)
validation_predictions = booster.predict(validation)
auc_score = roc_auc_score(y_val, validation_predictions)
mlflow.log_metric('auc', auc_score)
signature = infer_signature(X_train, booster.predict(train))
mlflow.xgboost.log_model(booster, "model", signature=signature)
# Set the loss to -1*auc_score so fmin maximizes the auc_score
return {'status': STATUS_OK, 'loss': -1*auc_score, 'booster': booster.attributes()}
# Greater parallelism will lead to speedups, but a less optimal hyperparameter sweep.
# A reasonable value for parallelism is the square root of max_evals.
spark_trials = SparkTrials(parallelism=10)
# Run fmin within an MLflow run context so that each hyperparameter configuration is logged as a child run of a parent
# run called "xgboost_models" .
with mlflow.start_run(run_name='xgboost_models'):
best_params = fmin(
fn=train_model,
space=search_space,
algo=tpe.suggest,
max_evals=96,
trials=spark_trials,
)
The individual runs will be displayed in the “Experiments” page.
Now we can select the top performing model, register it and a new version will be created.
best_run = mlflow.search_runs(order_by=['metrics.auc DESC']).iloc[0]
new_model_version = mlflow.register_model(f"runs:/{best_run.run_id}/model", model_name)
It’s time to use the optimized model for some actual predictions on new, unseen data.
# To simulate a new corpus of data, save the existing X_train data to a Delta table.
# In the real world, this would be a new batch of data.
spark_df = spark.createDataFrame(X_train)
table_name = f"{CATALOG_NAME}.`{SCHEMA_NAME}`.wine_data"
(spark_df
.write
.format("delta")
.mode("overwrite")
.option("overwriteSchema",True)
.saveAsTable(table_name)
)
Then we retrieve the newly created, optimized model and use it to generate predictions.
from pyspark.sql.functions import struct
apply_model_udf = mlflow.pyfunc.spark_udf(spark, f"models:/{model_name}/2")
new_data = spark.read.table(f"{CATALOG_NAME}.`{SCHEMA_NAME}`.wine_data")
# Apply the model to the new data
udf_inputs = struct(*(X_train.columns.tolist()))
new_data = new_data.withColumn(
"prediction",
apply_model_udf(udf_inputs)
)
The last column indicates whether a specific wine is considered to be high quality. A value above 0.5 could be interpreted as a high quality wine.
Databricks and MLflow - Our Conclusion
With this simple example of an end-to-end machine learning project, it is pretty evident that MLflow is a powerful tool at every data practitioner’s disposal. With an intuitive (and very Pythonic) API, few lines of code are sufficient to design and execute experiments, log metrics and results, store and retrieve models and keep track of their performance over time. Its seamless integration with Databricks, lets us leverage components such as Unity catalog for storage, a powerful Spark backend for complex calculations and a Jupyter notebook environment for developing and collaborating with others. In the ever-changing field of MLOps, tools and frameworks come and go regularly but few succeed in establishing themselves among engineers. Databricks is a great example of a data platform that its versatility and ease of use makes it an obvious choice for any machine learning project; small or large and especially when paired with MLflow, the results are impressive.
Do you have questions about MLflow, Databricks or other topics? If you would like to discuss your organizations’ specific needs, we are eager to learn about your challenges and happy to help finding and implementing optimal solutions.
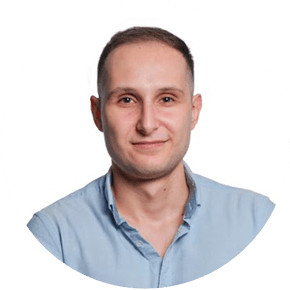