As digitalization advances, the amount of data that companies have to process is growing, along with the diversity of that data. In addition to structured data from ERP-systems, vast amounts of unstructured data in the form of documents or mass data from IoT systems are also being added. To remain competitive, the demand for a modern data platform architecture is growing. Such an architecture should enable seamless integration, scalability and advanced analytics using machine learning (ML) and AI.
Our Blog Series "Business Data Cloud" at a Glance
|
Therefore, more and more companies are turning to Lakehouse architectures that combine the advantages of data lakes and data warehouses. The Lakehouse approach provides a unified platform for structured and unstructured (mass) data that combines cost-efficient storage, high-performance queries, and integrated governance functions. As a basis for ML and AI applications, this architecture enables companies to make data-driven decisions, optimize processes, and develop new business opportunities.
In light of these trends, the question arises as to whether SAP Datasphere is up to these requirements as a data platform. Might other solutions such as Databricks come into play?
In this article, we explore the strengths and weaknesses of these two tools and analyze whether a combination of the two can create the foundation for such a modern data architecture.
Framework for evaluation
To ensure a consistent comparison, a structured framework is needed. Based on our years of experience, we have summarized the relevant criteria in the following overview. This represents the full range of functions of a modern data platform. Among other things, we evaluate the integration options of different source system classes, as well as the storage options and data processing options. In addition, data modeling and the subsequent use of the data also play an important role. The cross-sectional functions of a modern data platform are mapped in the form of governance, searchability, collaboration and security.
We have evaluated the functions for each solution and color-coded them using a traffic light system. Green indicates native, solid support for the functionality, while yellow indicates limitations. Cells marked in red indicate that the function is missing or only supported to a very limited extent. The following illustration shows our assessment of SAP Datasphere in this regard.
Strengths and weaknesses of SAP Datasphere
As you can see, SAP Datasphere covers the classic data warehouse components particularly well. As an SAP solution, Datasphere scores with strong integration with the SAP ecosystem, but also offers support for many non-SAP data sources. However, the latter sometimes comes at the expense of reduced delta capabilities. Event streaming is currently only supported in the form of an integration with Kafka.
To extend the functionalities, especially for more complex use cases, SAP is positioning its Business Technology Platform (BTP) with various sub-services as the go-to solution. However, this leads to design breaks and additional costs. SAP Open Connectors (part of the BTP Services Integration Suite), for example, enables the connection of RestAPIs to Datasphere, but leads to additional fixed and usage-based costs.
In terms of modeling, SAP Datasphere offers a sophisticated semantic model that has a strong integration with S/4 HANA in particular. Here, Datasphere also scores with a powerful self-service concept – even for non-technical users. When it comes to consuming data, SAP Datasphere benefits from its integration with SAP Analytics Cloud, making it ideal for dashboards and business planning.
SAP Datasphere offers the option of executing model training and predictions directly in the underlying SAP HANA Cloud Database with HANA Predictive Analysis Library (PAL) and Automated Predictive Library (APL). Since the calculations are performed directly on the database, there are significant performance advantages. There is no need for data transfer between client and server. However, support for machine learning applications is severely underdeveloped compared to competitors (e.g. Databricks). Among other things, there is no way to use arbitrary Python libraries. A data science & machine learning workspace with the corresponding functionalities is also not available.
Since December 2024, the Datasphere Object Store, which is the basis for a lakehouse architecture, has been in restricted release. This is a big step forward. Unfortunately, some important features are still missing to improve our rating here. For example, interoperability (i.e. the use of external object stores by Datasphere and the use of the Datasphere object store from external sources) is still poor or non-existent. Even within Datasphere, the object store is not well enough integrated to enable direct and high-performance reporting.
Watch the recording of our webinar
"SAP Datasphere and the Databricks Lakehouse Approach"
Strengths and weaknesses of Databricks
In contrast, Databricks offers good integration of data sources outside the SAP ecosystem. Extraction from SAP systems is currently being restricted by SAP and is to be carried out via SAP Datasphere.
Databricks allows the connection of any data source via Python program routines, so that all conceivable combinations of file formats, transmission protocols, authentication mechanisms and data modeling can be covered with dedicated programming if necessary.
Databricks is the pioneer and namesake in the area of data lakehouses. This architecture separates storage and compute resources, enabling both components to scale independently. Communication between storage and compute layers uses protocols and concepts that natively support parallelization and horizontal scaling. This way, much larger data volumes can be stored and processed much more efficiently than with traditional database systems.
In addition, Databricks is a mature and constantly evolving development platform for ML, deep learning, generative AI, retrieval augmented generation, agent systems and all other varieties of current data science trends.
Data storage, feature store and model registry are all integrated into the Unity Catalog (UC) as the central index of the Lakehouse platform. The associated open-source ML development framework mlflow, the de facto industry standard, is used for tracking experiments, training data, model versions, evaluation and usage metadata and is an original in-house development by Databricks.
Machine learning workflow and tool support in Databricks. Source: Databricks, 2025
Combined approach
Since the strengths of the two tools complement each other, combining them offers enormous potential. The following graphic shows the strengths of SAP Datasphere in blue, while the strengths of Databricks are shown in red. The integration should be evaluated based on the respective source system class. SAP Datasphere is convincing as a business layer in a standardized data warehouse approach. It also offers seamless integration with SAP systems and provides a powerful consumer layer for business intelligence and reporting with SAP Analytics Cloud. Databricks, on the other hand, is particularly strong in the area of machine learning and enables a data lakehouse architecture that can manage large amounts of data cost-effectively. By combining these strengths, companies can build a modern, scalable, and intelligent data platform.
Conclusion and outlook
In the future, data lakehouse architecture will continue to play a leading role in dealing with ever-growing data volumes and challenging ML & AI requirements. With the restricted release of the embedded object store, SAP has taken a step in the right direction, but will still find it difficult to catch up in these areas in the next few years. For companies that cannot or do not want to wait, the combination of Datasphere and Databricks is an optimal solution. For more details on this topic, watch the recording of our webinar "SAP Datasphere and the Databricks Lakehouse Approach - How to build a Future-Proof Data Platform".
We are excited to see how SAP responds to these challenges in the face of competition from rivals. Although it has been partnered with Databricks for some time, the results for the end customer have so far been sobering.
However, both sides have announced that they are working on something big. Will this weaken or strengthen the combined approach? We remain excited and will report.
Do you have questions about Databricks and SAP Datasphere? Please do not hesitate to contact us. We look forward to exchanging ideas with you!
Thanks to our Data Science and Engineering team for contributing their Databricks expertise to this article.
Machine Learning, SAP Data Intelligence, Datasphere
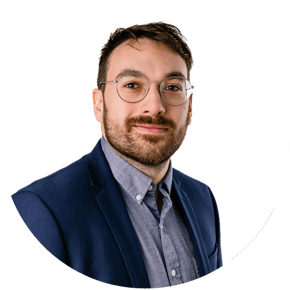